What kind of information do children and infants take into account when learning new words? And to what extent do they need to rely on interpreting a speakers intention to extract meaning? A paper by Morse, Cangelosi and Smith (2015), published in PLoS One, suggests that bodily states such as body posture might be used by infants to acquire word meanings in the absence of the object named. To test their hypothesis, the authors ran a series of experiments using a word learning task with infants—but also a self-learning robot, the iCub.
The word learning task used in this study was the Baldwin task: Two objects are presented to the infant multiple times. However, they are not named in the presence of the object. Instead the experimenter hides both objects in two buckets, then looks at one bucket and names the object (e.g. “Modi”). Then the two objects are taken out of their containers, put on a pile, and the child is asked to pick up the Modi. Children as young as 18-20 months do fairly well in this task, and their high performance has generally been interpreted as evidence that they have used a form of mind reading or mental attributions to infer which object is the Modi, as the object and the word do not appear simultaneously.
However, proponents of non-mentalistic approaches to language acquisition have come up with alternative explanations. For example, a previous study by Samuelson et al., (2011) has found that spatial location can greatly contribute to word learning in infants and in computer simulations using Hebbian learning. Hebbian learning is a form of associative learning which is loosely based on the way the neurons in our brain are assumed to learn as well. This very simple way of associative learning does not take into account the intention of the speaker at all, instead the model used by Samuelson et al. only takes into account the spatial location over time.
Morse et al. (2015) looked at the bodily state instead of the surrounding context. As in the simulation by Samuelsson et al., the iCub robots use simple associations to make sense of their environment and no mental representations.
Video of the iCub robot used by the team of Morse et al, replicating the study by Smith and Samuelson (2010). The iCub can learn objects that are in and out of sight through Hebbian associative learning.
They have three layers of “neurons”—one for the visual inputs, one for the motor inputs and the third for the word input. Within these fields, the actual connections and their weights are randomly assigned and only change with experience. The model works purely associatively and takes all available inputs into account—and if spatial or postural information are helpful, then that’s what the model uses.
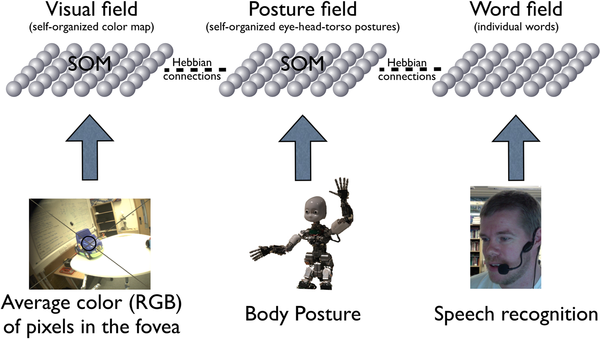
In addition to the original Baldwin task, Morse et al. added several variants: In the switch task (which was also used by Samuelson et al.), the location of the object changed after the was taken out of the container before the child was asked to choose the Modi. In the interference condition, the target object is visible to the child, however it is presented in the spatial location of the competitor object. Finally, Morse et al. manipulated the sitting position of the children. Whilst in the previous studies, the infants and iCub robots were either seated or standing throughout the entire trial, in the later trials, they would change their posture halfway through the trial.
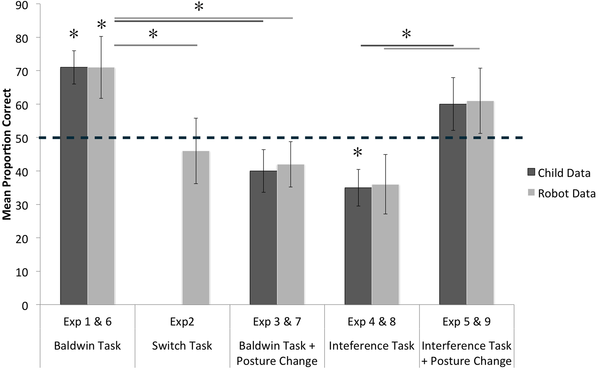
The results of infants and iCub robots closely match. In the original task, both robots and infants perform well above chance. However, in the interference task and the switch task, they are at chance level. in fact the infants in the interference task seem to be below chance, attributing the new word with the wrong object. The robot data is not very different. It mainly shows a generally higher variance across all conditions.
How did the posture change affect the acquisition of words then? The data suggests that it generally improved performance in the interference task: Robots and infants did much worse without the posture change, suggesting that posture can be a very useful guide in structuring the learning environment if it co-occurs with the structure of the learning task. However, in the original Baldwin task, the posture change actually impaired performance and infants and robots operated at chance level if they changed posture. Here, the non-matching experience had a detrimental effect.
It is not surprising that infants might use their own bodily states, e.g. when learning words like “sit”, “stand”, “lie down”, “bed”, “chair” or others. However, in other instances, it might be a much less reliable cue, e.g. when learning words, and might be inhibiting associations (e.g. as in the interference condition). So, whilst posture might make language acquisition easier, it might also make it harder if the cues do not neatly line up. However, such instances should be rare. Learning takes place within temporal and spatial contexts that remain the same throughout a learning episode, and parental speech is particularly repetitive. The interference condition is quite unnatural by inducing a change of posture mid-way, just before the new word is uttered.
Overall, given the model’s simple three layer design it should come as no surprise that posture does potentially affect word learning in the iCub. However, what is really nice about the data is that it closely matches infant performance across the variety of sub tasks. The results of both, robot and infant data, suggest that infants may no need to accurately represent the speaker’s intent when learning words, but are able to make the correct word-meaning associations based on visual and spatial information. Furthermore, they do not seem to use complex mental representations in the interference condition and the posture change tasks, in which the posture change has a detrimental effect on children’s word learning.
Whilst reading the article, I kept wondering what the advantage of using the iCub are and whether the results would look different if a simple computer model was used—which is a common objection to iCub research. However, unlike a computer model, the robot model would be exposed to similar levels of noise compared to the infant. The question is—to what extent does this noise make the results more or less comparable to the original data? In Morse et al., the outcomes seemed to match closely, but how would it have looked if a simulated neural network would have been used without the robot? By glancing at the data by Morse et al. (iCub robots) and Samuelson et al (Simulations), the main difference between simulations and the iCub is that the iCub data has higher standard deviations for a larger number of subjects (20 trials/condition) compared to the infants, but the simulations (12 simulations/condition) have lower variance. However, this is no formal analysis and it generalises across similar but different tasks. Still, two questions remain: If, on the one hand, we would get considerably different results, which one is more informative? If, on the other hand, the results are largely the same, what’s the point in getting a costly robot to do the task?
The robots seem to be quite cool to play around with. But more importantly, the results of the iCub study by Morse and colleagues and the previous simulation by Samuelson and colleagues show that infants may not need to engage in thinking about speaker intentions when learning a language. Instead, they may be getting much further using spatial and posture information to make sense of the input. This does not prove that the social theory of language acquisition is off the table though: Infants still need to pay attention to social cues and may use mentalising in other contexts.
References
Baldwin, D. A. (1993). Early referential understanding: Infants’ ability to recognize referential acts for what they are. Developmental Psychology, 29(5), 832–843. doi: 10.1037/0012-1649.29.5.832
Morse A.F., Benitez V.L., Belpaeme T., Cangelosi A., Smith L.B. (2015) Posture Affects How Robots and Infants Map Words to Objects. PLoS ONE 10(3): e0116012. doi: 10.1371/journal.pone.0116012
Samuelson L.K., Smith L.B., Perry L.K., Spencer J.P. (2011) Grounding Word Learning in Space. PLoS ONE 6(12): e28095. doi: 10.1371/journal.pone.0028095
Many thanks to the attendees of the Babylab Journal Club at Lancaster University, in particular Katherine Twomey, for the discussion and suggestions.
Great post. I’ve linked to it here: http://new-savanna.blogspot.com/2015/10/posturing-robots-and-infants-contra.html